Full-length self-paced online courses from Mike X Cohen
Some of my courses are self-hosted; you don't need a coupon because I don't play algorithmic games with course prices.
When enrolling in my Udemy courses, use the coupon code "202506" for the maximum discount that Udemy allows. That coupon is embedded in all links on this page.
A deep understanding of deep learning
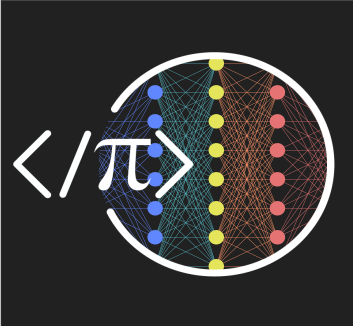
57+ hours of instruction on modern deep learning (using PyTorch), with LOTS of exercises and code-challenges to help you hone your DL skills. Includes a Python tutorial.
Statistics and machine learning
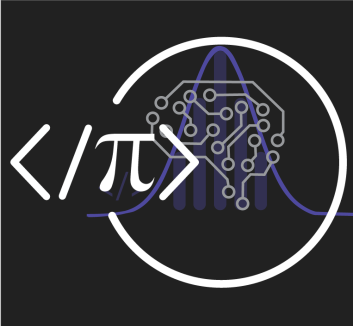
35 hours of instruction (>50 hours in total, including exercises) on a rigorous deep-dive into statistics and machine learning. All concepts are implemented in Python and MATLAB.
Improving data quality in data analytics
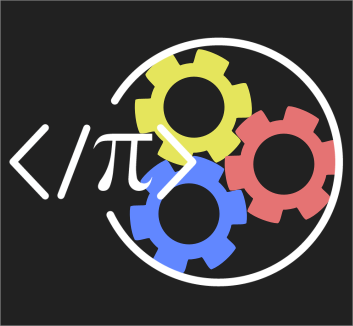
Learn why, when, and how to maximize the quality of your data to optimize data-based decisions.
Basic statistics and data literacy
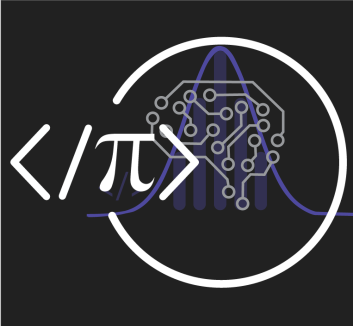
Free short course on statistics lingo and concepts (no math or code!).
Mathematics: learn the language of science
Master math by
learning Python
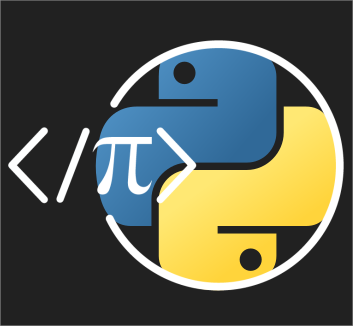
37+ hours of instruction (>60 hours in total, including exercises) on how to use Python as a tool for learning concepts and visualizations in mathematics.
Linear algebra:
theory and code
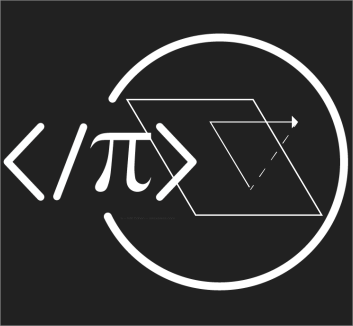
30+ hours of clear explanations of concepts in linear algebra, including vectors, matrix multiplications, least-squares projections, eigendecomposition, and singular value decomposition.
Master calculus 1
using Python
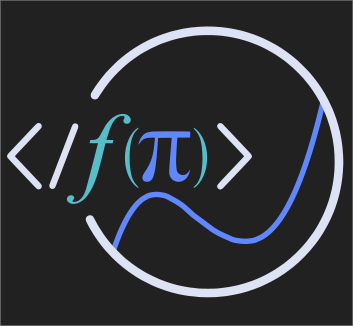
Over 41 hours of instruction (including a Python intro tutorial). Develop a deep understanding and intuition for calculus. Solve problems and implement algorithms by hand and in Python.
Master calculus 2
using Python
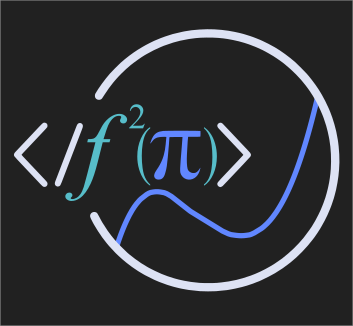
Over 19 hours of instruction. Extent your calculus skills with integration and numerical approximations.
Generative AI for academic and scientific applications
Generative AI for data analytics
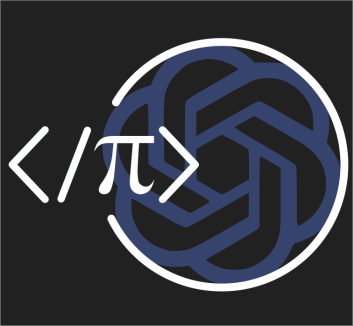
4+ hours on using ChatGPT to code, learn about, apply, and communicate data science. If you're new to ChatGPT, this course will change your life.
ChatGPT for academic and scientific writing mastery
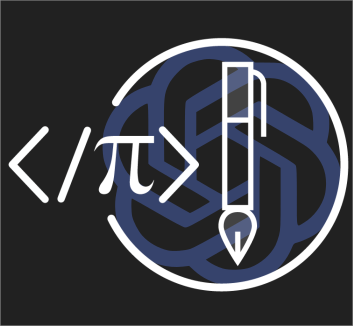
3.5 hours of clear instruction on how to use AI-assisted writing for various tasks. The goal is to use ChatGPT to help you be a better writer.
Unleash your math potential with ChatGPT
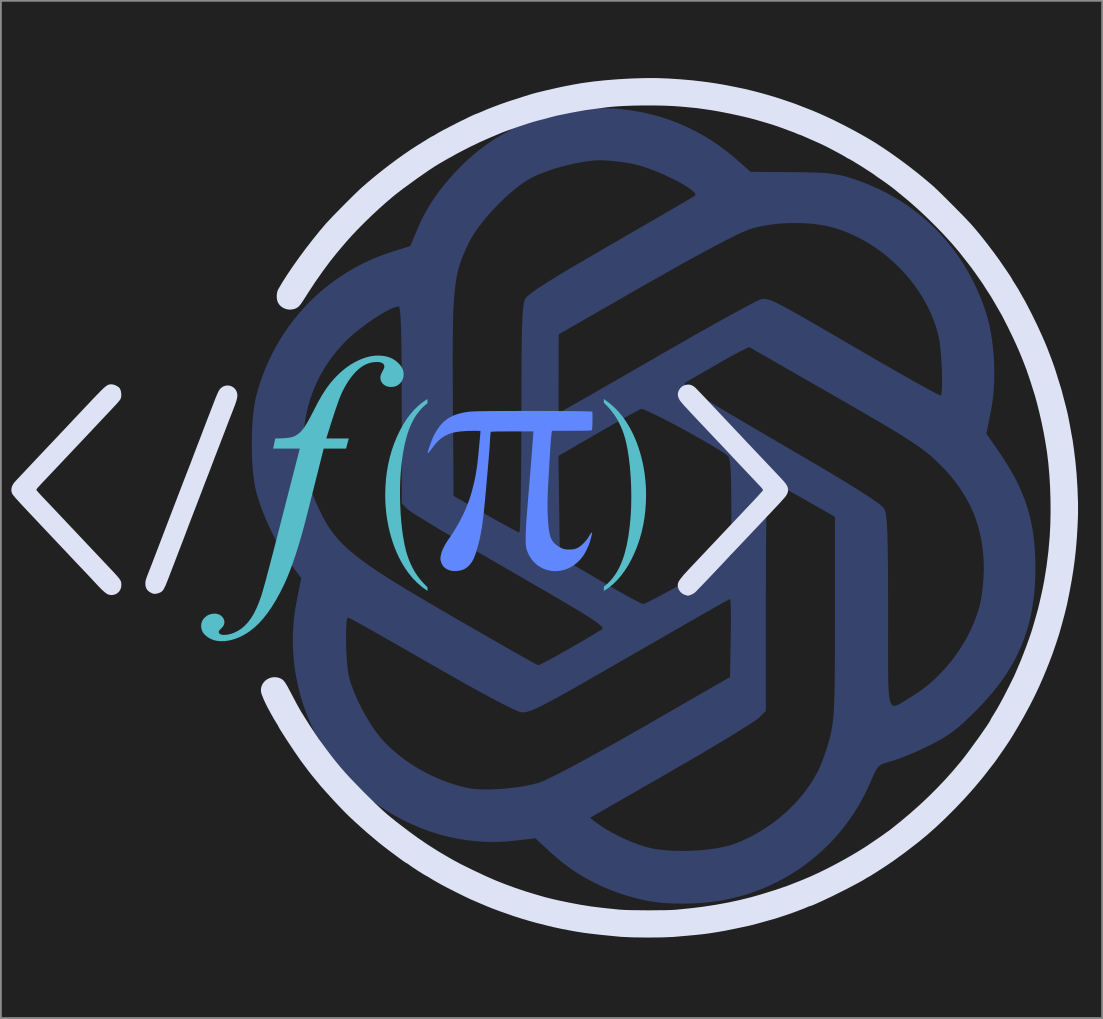
Learn how to use ChatGPT to accelerate your math and quantitative reasoning skills.
Signal processing: analyze and visualize time series data
Solved signal processing problems
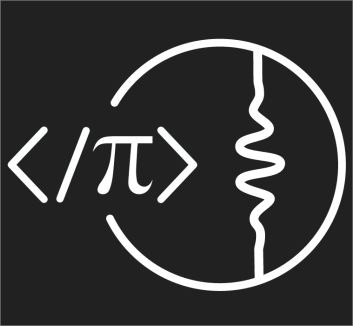
12+ hours of instruction about applied signal-processing methods, including filtering, denoising, convolution, resampling, interpolation, and outlier detection.
Understand the Fourier transform
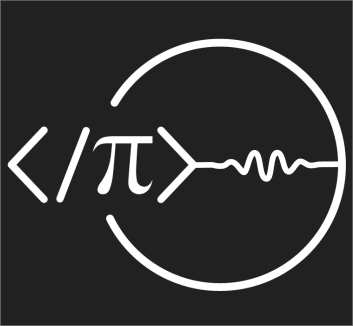
Theoretical and computational bases of the Fourier transform, and its implementation in modern applications in digital signal processing, data analysis, and image filtering. MATLAB and Python codes are included.
Solved challenges in neural time series analysis
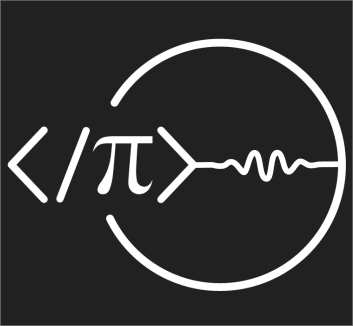
45+ hours of video lectures that supplement my book "Analyzing Neural Time Series Analysis." Includes a lot of exercises with fully explained solutions.
PCA & multivariate signal processing
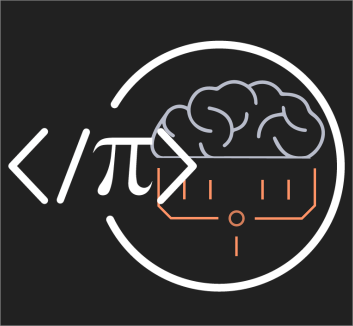
17 hours of instruction on theory and implementation of dimension-reduction and source-separation methods for multivariate data. Includes MATLAB codes, sample data, and a 3-hour linear algebra crash-course.
Learn Python for science and data applications
Master Python for scientific coding
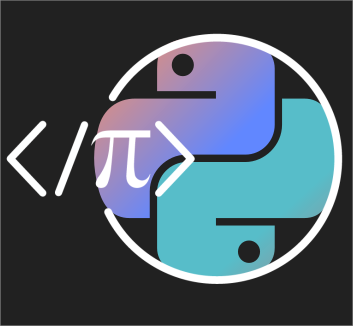
32+ hours of instruction (>80 hours in total, including exercises) on using Python for scientific computing. No previous background in Python necessary!
Master math by
learning Python
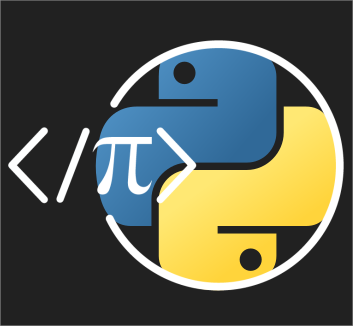
37+ hours of instruction (>60 hours in total, including exercises) on how to use Python as a tool for learning concepts and visualizations in mathematics.
Learn MATLAB: the language of technical computing
MATLAB onramp: Learn scientific coding with ChatGPT's help
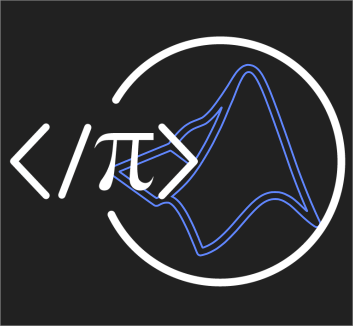
6+ hours of video instruction on getting started with MATLAB, debugging code, and developing a programming style. Learn how ChatGPT can help you learn MATLAB. Ideal for a beginner who needs to get up-to-speed with MATLAB.
Master MATLAB through guided problem-solving
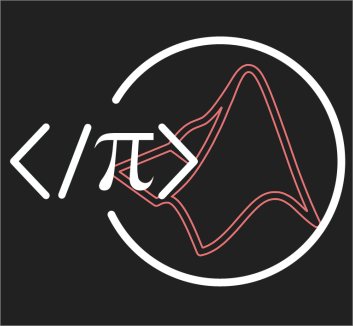
40 hours of video instruction on MATLAB programming instruction (longer if you do all the exercises!). Lessons revolve around specific applications in graphics, data analysis, image processing, import/export, and so on.
Essentials of MATLAB for neuroscience
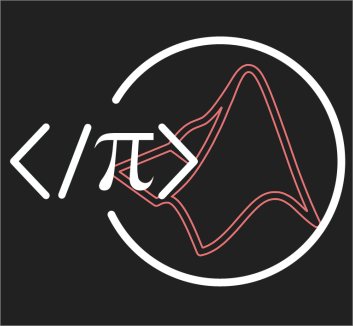
This course teaches MATLAB programming in neuroscience data analysis using MATLAB. Each of five modules covers a range of specific data processing, analysis, and visualization skills that neuroscientists often need.